Blog
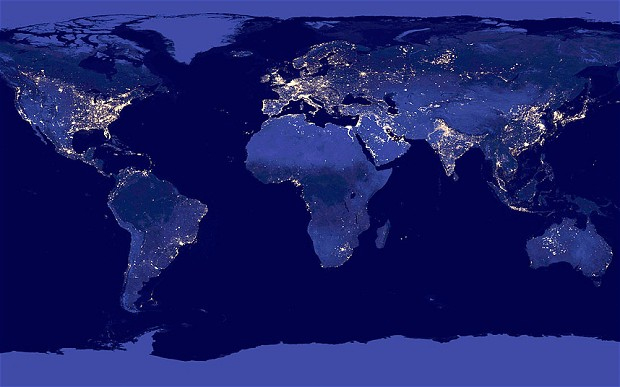
A recent ISEP publication (forthcoming in the International Journal of Remote Sensing) details both the promise, as well as some of the likely pitfalls, of using satellite imagery data for generating data on energy access. The study is, by far, the largest and most geographically diverse study of the popular night lights satellite dataset. It reveals that these satellite imagery datasets can produce reliable measures development outcomes specifically related to the phenomenon the satellites observe (electrification in this case), but struggle as measures of other outcomes (like capital accumulation). It also demonstrates that the utility of the data depends substantially on supporting data (like the quality of map data) and on the level of development in the area of study. These are all important lessons in the coming satellite data revolution.
Many of the people who will be reading this blog post can still remember the revolution that took place in the 1980s and 90s with personal computing. Prior to this time, if someone wanted to run a complicated simulation or statistical analysis, they had to have access to one of the large mainframe computers, whose size and expense made them primarily the property of governments, universities and large corporations. The introduction of microprocessors and personal computers changed this dramatically – today’s elementary school student often has access to more computing power in their home than many energy professionals used to have at work.
We may be experiencing a similar process today with regards to satellite technology. Two decades ago, Bob Twiggs was teaching at Stanford University and challenged his students to design a satellite, but, in order to make it more challenging, he forced them to design a system that would fit into a 4-inch Beanie Baby box (for those of you who don’t remember the Beanie Baby craze, here is the link). Thus was born the picosatellite – a small, low cost, satellite that could be utilized for a variety of purposes.
Today there are a plethora of projects attempting to get smaller satellites into orbit. Planet, for example, is a private company that utilizes a chain of satellites constantly orbiting the earth to collect high resolution pictures of the planet at all times. From this information, they design computer algorithms to monitor supply chains, natural disasters and a variety of other metrics that may interest other companies. Everyone from NASA to SpaceX is now trying to encourage the development and deployment of smaller and smaller satellites that can do everything from monitoring pollution to creating an artificial meteor shower.
But what does all of this mean for energy research? Can the potential from this data really live up to the hype? Eugenie Dugoua (Columbia University), Johannes Urpelainen (Johns Hopkins, SAIS) and I approached a specific part of this question in our forthcoming article in the International Journal of Remote Sensing. One of the best known publicly available satellite imagery datasets is the night light dataset. Originally designed by the military to spot cloud-cover at night, the satellites could be used to stitch together a composite of the intensity of lights on the ground around the world. One of the most famous examples of this analysis is the picture of North and South Korea at night, which illustrates dramatically the differences in economic development between the two states.
The advantages to this data are numerous. First, it allowed for analysis on a scale not previously possible. A pixel from the night lights dataset is about 1km square. This can allow analysts to look at sub-national and village-level trends in electrification. Second, it addressed the issue of missing or unreliable data. Some governments only report data where it looks positive or is easy to gather (like North Korea’s economic data), but the satellites do not select their data on the basis of politics.
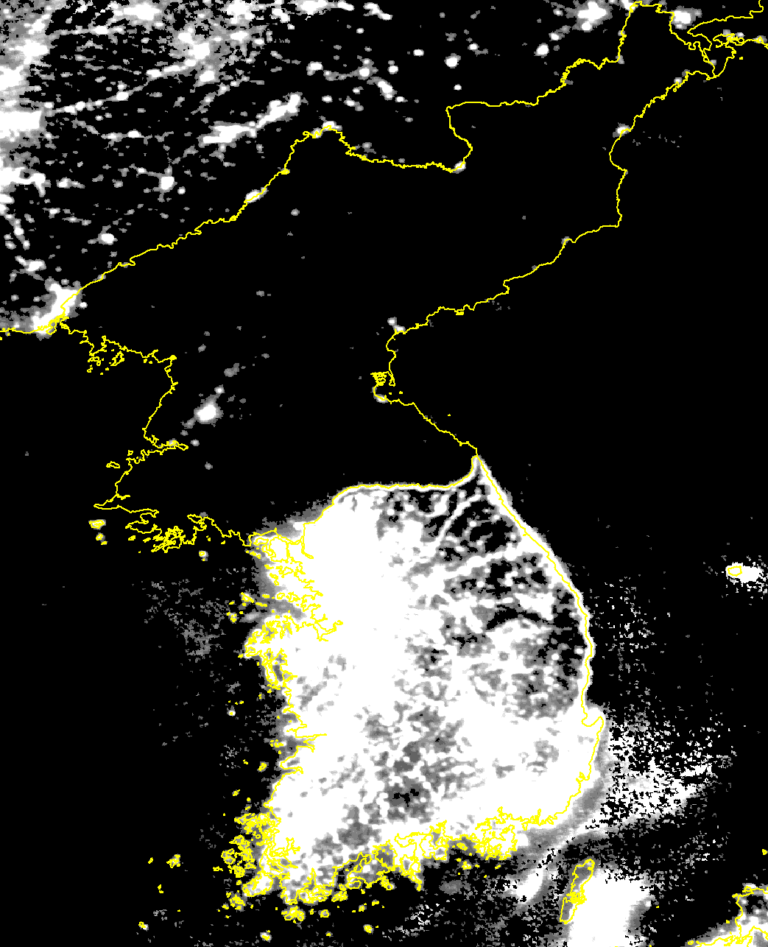
Because of these advantages, the night lights data has been used to evaluate all kinds of issues, from election-time manipulation of the economy to proxying economic statistics.
Yet, there have been very few validations of the data on the sub-national level, and those that had been done were relatively small and relied on inconsistent sampling. We evaluated the night lights data against a comprehensive dataset of electrification in villages in India. The results suggested several important conclusions:
- While the night lights data did a reasonable job of capturing differences in rural electrification, the methods utilized made a substantial difference in how well it performed.
- The performance of night lights to detect electrification also depended greatly on the availability of geographic data for villages — where we had only the latitude and longitude of the village, night lights performed much worse than when we actually had the shape of the village.
- Between states in India there was substantial variation in how well the Night Lights data performed as a proxy for electrification. As the Figure from our paper shows (reproduced below), this is related to the quality of electricity supply. This suggests that Night Lights may perform better in more developed areas.
- Night lights did a worse job as a proxy for economic status of the villages, especially as it related to capital resources like banking.

These results have implications for the oncoming satellite revolution. While exciting, the ability to use data from these new satellites will still be dependent on our ability to conduct careful validation of the data we derive. As with the hype around “big data”, it is far too easy for project managers in both the public and private sector to latch onto the potential of these monitoring systems before their utility is firmly established.
We need to also be aware of the extent to which the use of satellite data resources still depends on traditional data collection. If, for example, we wanted to identify particular types of power plants from satellite pictures, we would still need a set of labeled plants on which we can train a computer to make this identification. As we found out, the success of satellite data relied heavily on simply having good maps.
Finally, we have to be careful about understanding the context of satellite data. In our case, India has made very large investments into electrification in rural and poor areas of the country. This helps to explain why the night lights data was a relatively good proxy for electrification, but not as good of a proxy for economic development. Similar patterns are likely to be observed with other types of data.
As we move into an exciting new period of satellite technology, it is useful to be reminded that these are not magical instruments and that data almost never “speaks for itself.” ISEP looks forward to assisting in the development and effective analysis of data during the satellite revolution.